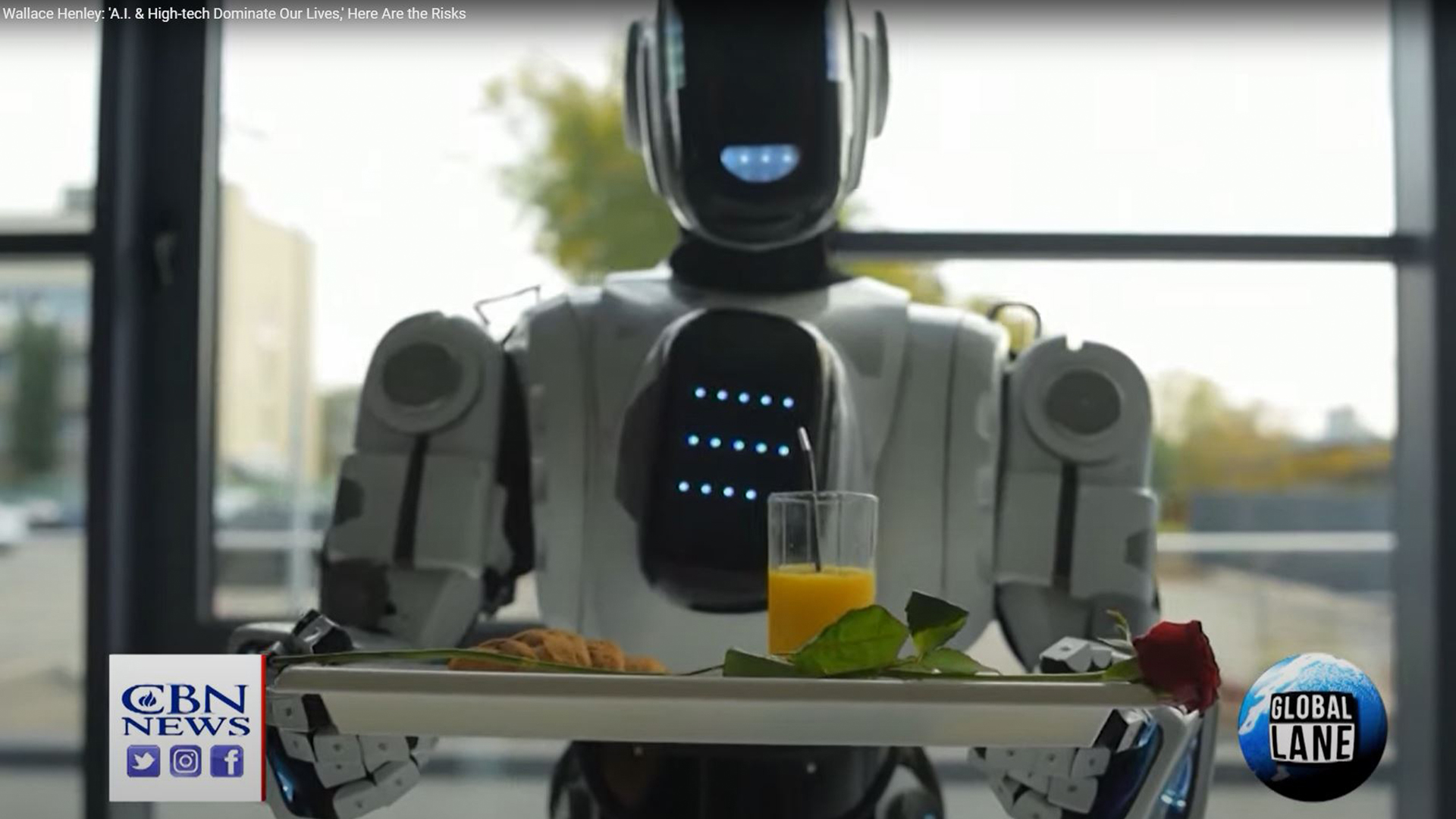
Deep learning for regression is something that you have probably heard of. Deep learning for regression can be a powerful new technology. It can predict the weather and even find out what your children eat for breakfast. But how does it apply to regression? Let's look at the main principles behind deep learning to predict regression. There are many types of deep learning. There are two methods that can be used to achieve deep learning.
Less-squares regression
There are two types of least-squares regression procedures: mathematically simple ones that place many restrictions on the input data and mathematically complex ones that put few restrictions on the data. The former is easier to learn from small data sets, but it can be more difficult to use and detect mistakes. When possible, you should use simpler procedures. Here are some least-squares procedures.
Also known as the Residual Sum Squares, Ordinary least squares can also be called the Residual Sum Squares. It is a form of optimization algorithm, in which an initial costs function is used to increase/ decrease the parameters till a minimum is reached. This method assumes that sampling errors are normal. But, the method can still work even if the distributions of samples are not normal. This is a common limitation for least-squares.
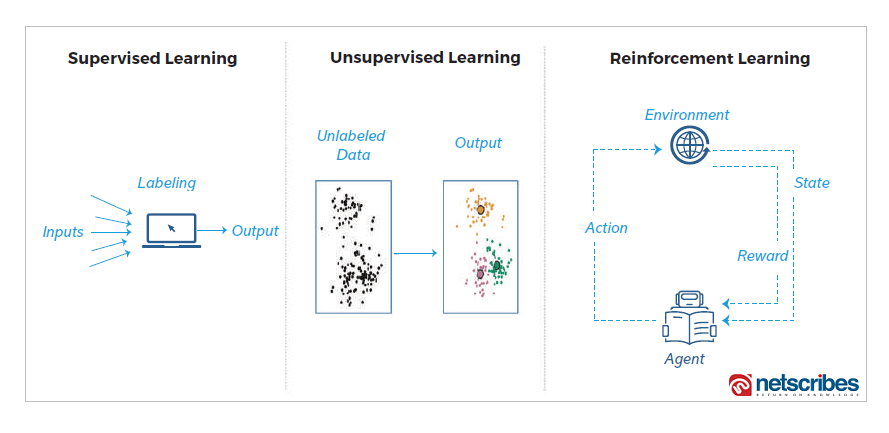
Logistic regression
Logistic regression, a statistical technique used in predictive analytics and data science to predict the likelihood that a particular outcome will occur based on input data. Logistic regression works in the same way as other supervised machinelearning models. This method classifies inputs into a binary category or multinomial one to predict trends. A binary logistic regression model for cancer can, for instance, identify someone who is high-risk compared to someone who is lower-risk.
This method can be used to predict whether someone will pass or fail a test based on their score. A student who studies for one hour per day might score 500 points more than someone who studies three hours per day. If the student studies for three hours per days, then the chance of passing the test is zero. With logistic regression, however, the model is not as accurate.
Support vector machines
Support vector machines (SVMs) are widely used in the field of statistical machine learning. These algorithms are built on a kernel-based approach. These algorithms are extremely flexible, versatile and adaptable. This is important for some types of applications. This article will examine the benefits of SVMs when it comes to regression. These models have many key features. We'll be discussing them here. Let us begin with the most commonly used examples to show how they work.
Support vector machines have a high level of effectiveness when working with large datasets. These models, unlike other forms of machine learning require a very small number of training points. Because they can use multiple kinds of kernel functions, these models are memory-efficient. You can also specify the decision function as either custom or common. It is important to avoid over-fitting when selecting the kernel function. SVMs are best suited for small sample sets and require extensive training.
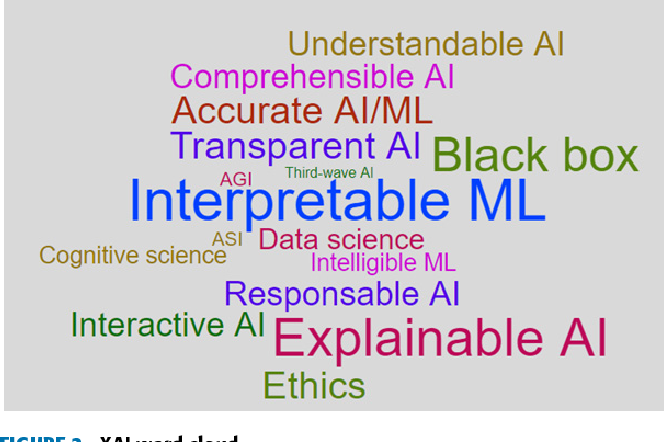
KNN
KNN is also known as lazy learning or instance-based learning. This type of algorithm does not require any prior knowledge or assumptions about the data. Because of this, it is suitable for classification and regression problems. The KNN algorithm is highly versatile and can be applied to a variety of real-world datasets. However, it is slow in fast-paced prediction environments and ineffective.
KNN algorithms use a number of examples in close proximity to predict a numerical value using data. This algorithm can be used for evaluating the quality and quantity of films by adding together the values from k instances. The K value is usually averaged from the neighbors. However, the algorithm can also use weighted median or average. The KNN algorithm can be used to predict images from thousands of pictures once it has been trained.
FAQ
What can you do with AI?
AI serves two primary purposes.
* Prediction – AI systems can make predictions about future events. A self-driving vehicle can, for example, use AI to spot traffic lights and then stop at them.
* Decision making – AI systems can make decisions on our behalf. As an example, your smartphone can recognize faces to suggest friends or make calls.
What is the current status of the AI industry
The AI industry is growing at an unprecedented rate. Over 50 billion devices will be connected to the internet by 2020, according to estimates. This will mean that we will all have access to AI technology on our phones, tablets, and laptops.
Businesses will have to adjust to this change if they want to remain competitive. Businesses that fail to adapt will lose customers to those who do.
Now, the question is: What business model would your use to profit from these opportunities? What if people uploaded their data to a platform and were able to connect with other users? Maybe you offer voice or image recognition services?
No matter what you do, think about how your position could be compared to others. Even though you might not win every time, you can still win big if all you do is play your cards well and keep innovating.
How does AI work?
An artificial neural networks is made up many simple processors called neuron. Each neuron receives inputs from other neurons and processes them using mathematical operations.
Neurons can be arranged in layers. Each layer has its own function. The first layer receives raw information like images and sounds. It then sends these data to the next layers, which process them further. The last layer finally produces an output.
Each neuron has a weighting value associated with it. When new input arrives, this value is multiplied by the input and added to the weighted sum of all previous values. If the result is more than zero, the neuron fires. It sends a signal along the line to the next neurons telling them what they should do.
This is repeated until the network ends. The final results will be obtained.
Who was the first to create AI?
Alan Turing
Turing was born in 1912. His father was a clergyman, and his mother was a nurse. He was an excellent student at maths, but he fell apart after being rejected from Cambridge University. He learned chess after being rejected by Cambridge University. He won numerous tournaments. He was a British code-breaking specialist, Bletchley Park. There he cracked German codes.
He died on April 5, 1954.
John McCarthy
McCarthy was born in 1928. He studied maths at Princeton University before joining MIT. There, he created the LISP programming languages. He had laid the foundations to modern AI by 1957.
He died on November 11, 2011.
Which countries are leaders in the AI market today, and why?
China is the leader in global Artificial Intelligence with more than $2Billion in revenue in 2018. China's AI industry is led by Baidu, Alibaba Group Holding Ltd., Tencent Holdings Ltd., Huawei Technologies Co. Ltd., and Xiaomi Technology Inc.
China's government invests heavily in AI development. The Chinese government has set up several research centers dedicated to improving AI capabilities. These centers include the National Laboratory of Pattern Recognition and the State Key Lab of Virtual Reality Technology and Systems.
China is also home to some of the world's biggest companies like Baidu, Alibaba, Tencent, and Xiaomi. All these companies are actively working on developing their own AI solutions.
India is another country that is making significant progress in the development of AI and related technologies. India's government is currently focusing its efforts on developing a robust AI ecosystem.
Statistics
- While all of it is still what seems like a far way off, the future of this technology presents a Catch-22, able to solve the world's problems and likely to power all the A.I. systems on earth, but also incredibly dangerous in the wrong hands. (forbes.com)
- By using BrainBox AI, commercial buildings can reduce total energy costs by 25% and improves occupant comfort by 60%. (analyticsinsight.net)
- Additionally, keeping in mind the current crisis, the AI is designed in a manner where it reduces the carbon footprint by 20-40%. (analyticsinsight.net)
- A 2021 Pew Research survey revealed that 37 percent of respondents who are more concerned than excited about AI had concerns including job loss, privacy, and AI's potential to “surpass human skills.” (builtin.com)
- More than 70 percent of users claim they book trips on their phones, review travel tips, and research local landmarks and restaurants. (builtin.com)
External Links
How To
How to setup Siri to speak when charging
Siri can do many different things, but Siri cannot speak back. This is because there is no microphone built into your iPhone. Bluetooth is the best method to get Siri to reply to you.
Here's a way to make Siri speak during charging.
-
Select "Speak When Locked" under "When Using Assistive Touch."
-
To activate Siri, double press the home key twice.
-
Siri will respond.
-
Say, "Hey Siri."
-
Simply say "OK."
-
Say, "Tell me something interesting."
-
Speak out, "I'm bored," Play some music, "Call my friend," Remind me about ""Take a photograph," Set a timer," Check out," and so forth.
-
Speak "Done."
-
If you wish to express your gratitude, say "Thanks!"
-
If you have an iPhone X/XS (or iPhone X/XS), remove the battery cover.
-
Reinsert the battery.
-
Assemble the iPhone again.
-
Connect the iPhone and iTunes
-
Sync the iPhone
-
Switch on the toggle switch for "Use Toggle".